Navigating creative destruction and potential diminishing returns in AI infrastructure
New AI research is being published every day and compounding at an unprecedented pace. How can one filter signal from noise? Are we fast approaching a plateau?
Two weeks ago, I spoke on a “Navigating the AI Investment Landscape” panel at Startup Grind Women’s Summit 2024, where we had a lively conversation around trends that founders should look out for in today’s AI fundraising environment.
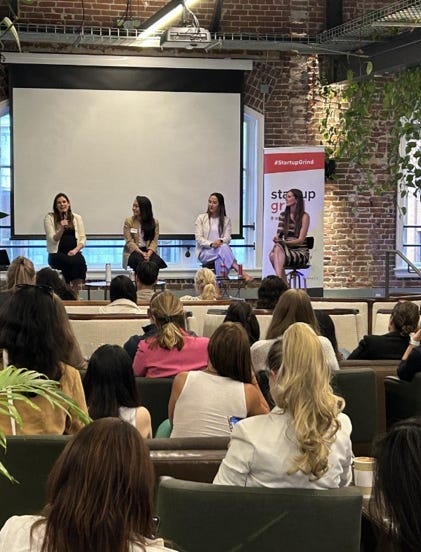
One of the key discussions was centered on evaluation approaches that VCs are taking to filter the signal from the noise, or “how to recognize AI snake oil” as Professor Arvind Narayanan of Princeton would say, since many companies are now emphasizing an AI narrative in their pitch. As an investor focused on the AI systems and infrastructure layer, this is a question I’m constantly asking myself, since we’re in an incredibly unique period where new AI research is being published every day and compounding at an unprecedented pace:
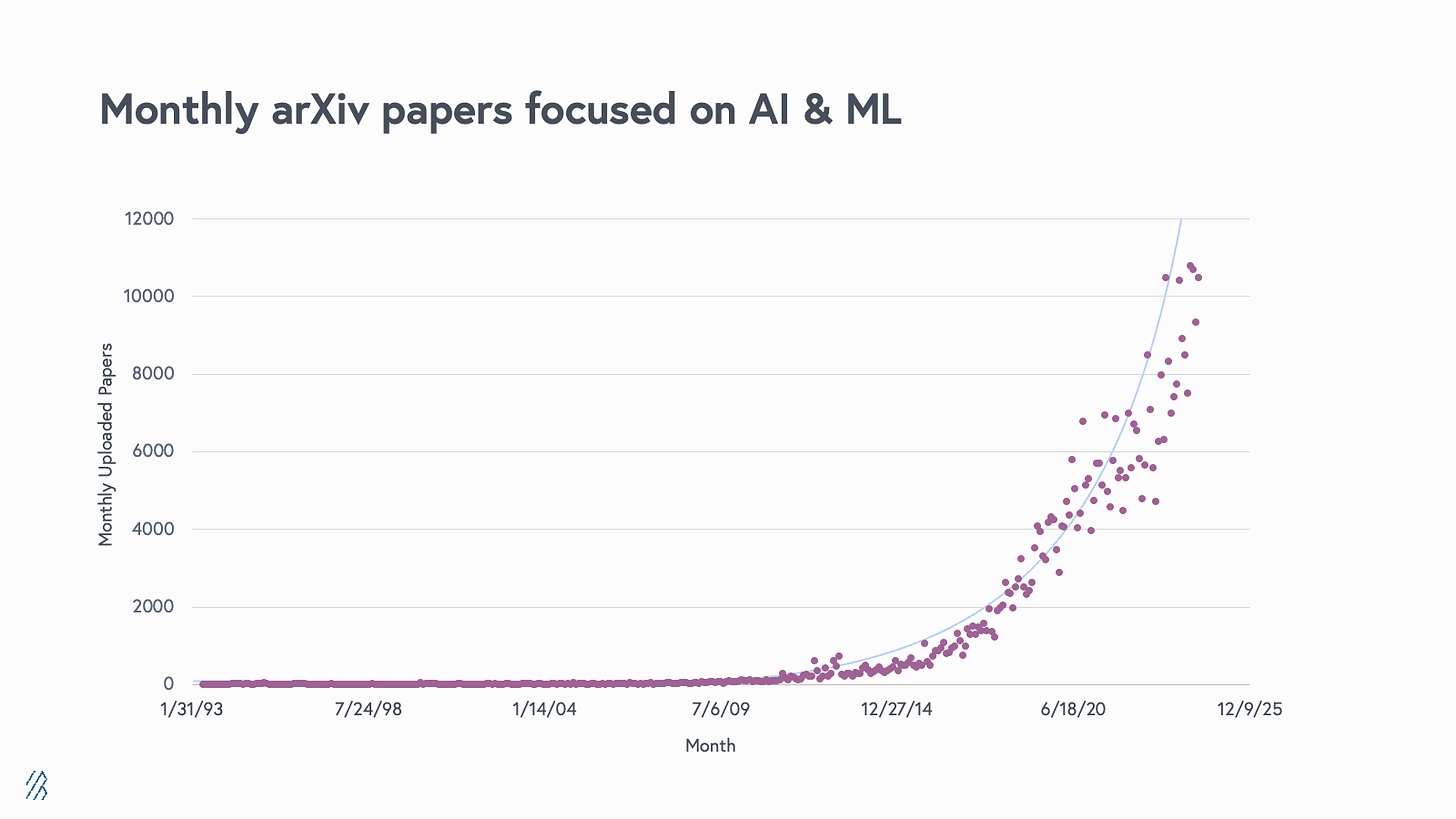
From cutting-edge ensemble methods to novel agentic approaches, new training techniques are being developed at breakneck speed. Alongside innovations in scaling laws, this progress is pushing the limits across all aspects of LLM performance and emergent abilities (chart below). Existing methods aren’t staying static either and are constantly evolving; just think about how many variants of RAG and CoT there are now! There are even audacious teams driven to debunk prior research and innovating on aspects that were previously thought immutable. As a result, the AI infrastructure stack is dynamically being defined and re-defined, which is extremely exciting to see, but also a reminder that investors need to tread carefully.
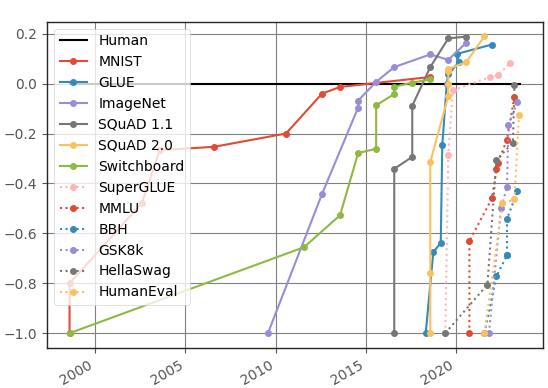
These are my long-form notes on three reflections that I shared during the panel from an investor’s perspective on how to navigate the current AI fundraising landscape, discussing examples in relation to companies that are commercializing AI model training and development techniques:
Prosecuting beyond the typical first-order questions
Rethinking first mover “advantage”
Bundling, unbundling, and re-bundling
1. Prosecuting beyond the typical first-order questions
When doing diligence on opportunities in the AI infra world, answering fundamental “table-stakes” questions (such as those around founder-market fit, team, market size, product love, etc.) is not enough. Rather, it is important to push toward a multifactorial framework, incorporating second-order questions such as:
Does this technique have longevity or is it a fad? Actual numbers can sometimes hide the full long-term story when taken point in time.
If there is longevity, will this become a primary technique or more related to niche use cases? Said another way, will enterprises default to this technique or will they only adopt this technique if other options are exhausted?
If it does have potential to become mainstream, where does value accrue in the stack? An example here would be how value has accrued to database companies rather than technique creators in certain situations.
Within the part of the stack where value accrues, is said company then well-positioned to capture value (especially in relation to the competitive landscape and in-house tooling using open-source options)?
2. Rethinking first mover “advantage”
Economist Joseph Schumpeter coined the term “creative destruction”, which describes the process where new innovations replace existing ones and render them obsolete over time. As highlighted earlier, we’re certainly living in a rapidly changing AI infrastructure paradigm where there is a high degree of creative destruction at play due to the fast pace of new research and evolving methods. This dynamic could diminish potential first mover advantage or any perceived “head start”. Imagine having invested millions into training a model using a specific parameter-efficient fine-tuning technique, only to have more efficient or superior techniques released a few months later (just check out Expert-Specialized Fine-Tuning as an example)!
I believe that everyone in the ecosystem, investors and founders alike, always need to anticipate any potential existential threat from base models getting better and better in the future. The rate of algorithmic progress on this front has been staggering: researchers estimate that the level of compute needed to achieve a given level of LLM performance has halved roughly every 8 months, which is substantially faster than hardware gains per Moore’s Law. Such rapid progress within foundational LLMs could very quickly overshadow the need for specific techniques and methods.
3. Bundling, unbundling, and re-bundling
The venerable Jim Barksdale once said “there’s only two ways I know of to make money: bundling and unbundling”. As investors, we must heed the wisdom that markets are constantly bundling, unbundling, and re-bundling — no industry is static.
Here are a few examples from markets that I spend time in. Just two years ago, the data community erupted with debate about unbundling and bundling dynamics within the modern data stack following Gorkem Yurtseven’s viral memo on the Unbundling of Airflow (here is a solid summary of “Bundlegate” in case you missed the ensuing discussion from 2022). In another example, the SaaS industry has gone through cycles of consolidation as well based on enterprise purchasing preferences toward end-to-end platforms vs best-in-class point solutions, and platform solutions tend to be more resilient during market downturns:
It’s common to see a highly fragmented landscape during the early innings of a new tech cycle as categories are being created, startups enter the market, and leaders emerge. Having just published Bessemer’s AI Infrastructure roadmap, one could infer that we are at this stage just by looking at the astounding number of logos featured in our market map:
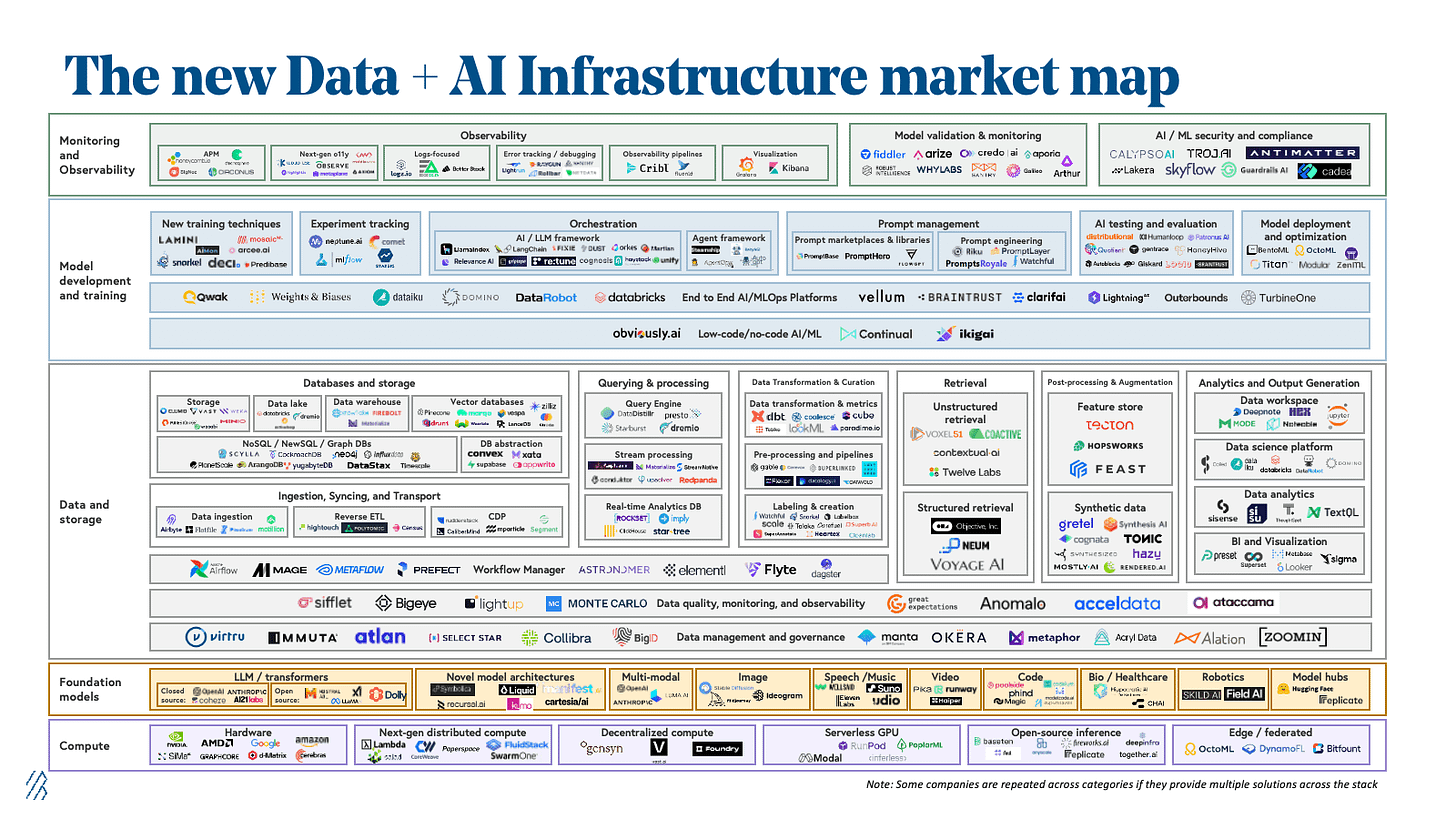
This map is constantly being updated due to the fast pace of AI progress, but at some point, one can plausibly assume that the number of logos will start to shrink rather than expand. Each time I revisit the map, I like to think about when and where consolidation could occur, and what thesis to pursue in each scenario. To complicate matters further, you’ll notice that public and scaled companies are present in our AI infrastructure market map. Unlike in previous tech shifts, many incumbents including Big Tech and Cloud Giants have been quick to participate and innovate alongside startups in the current AI infrastructure revolution. The active involvement of incumbents adds another layer of complexity to unbundling, bundling, and re-bundling dynamics since each incumbent runs their own unique integration vs modularization strategy that can have outsized impact on the landscape.
Are we reaching a plateau?
Innovation is happening so quickly that Leopold Aschenbrenner, a former member of OpenAI's Superalignment team, went viral last month for asserting that “AGI by 2027 is strikingly plausible” based on “trendlines in compute (~0.5 orders of magnitude or OOMs/year), algorithmic efficiencies (~0.5 OOMs/year), and “unhobbling” gains (from chatbot to agent)”:
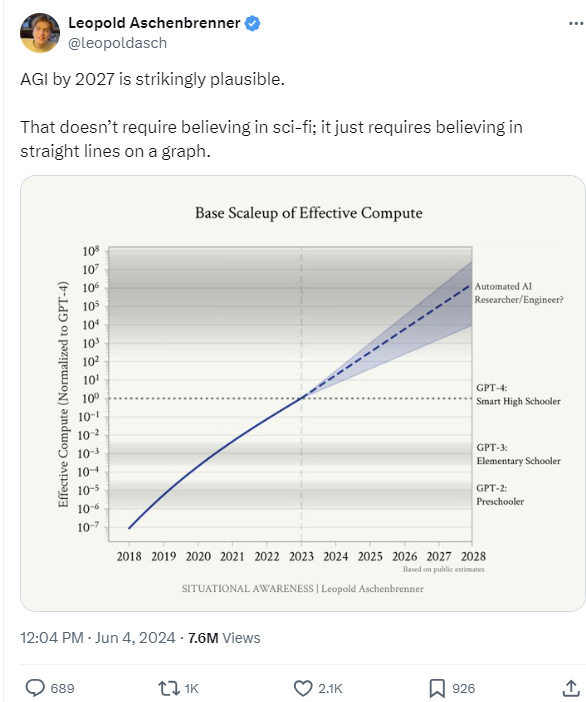
Presumably, the rapid pace of progress cannot be sustained forever, which begs a very important question: when might we hit a plateau? This has been a widely debated topic recently with AI thought leaders leaning in from all angles. A perspective from Professor Ethan Mollick of The University of Pennsylvania states that:
“As long as the scaling laws of training Large Language Models continues to hold, the way it has for several years, this rapid increase is likely to continue. At some point, development will hit a wall because of increasing expense or a lack of data, but it isn’t clear when that might happen, and recent papers suggest that many of these obstacles might be solvable. The end of rapid increases could be imminent, or it could be years away. Based on conversations with insiders in several AI labs, I suspect that we have some more years of rapid ability increases ahead of us, but we will learn more soon.”
Others like Princeton’s Professor Arvind Narayanan take a much different view, asserting that there is no empirical regularity to build confidence that the trends (such as around scaling laws) that have held previously should continue for many more orders of magnitude. Professor Gary Marcus of NYU goes one step further to present evidence that we may be fast approaching a plateau as LLMs are no longer advancing exponentially:
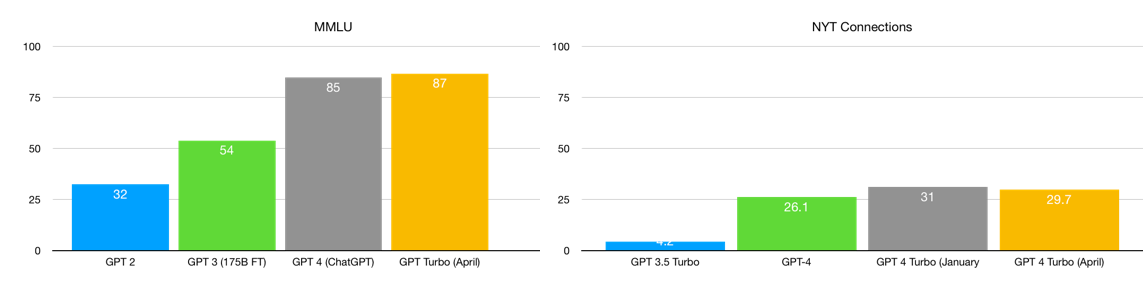
My take is that even if scaling laws are reaching a point of diminishing returns, this should give more drive to innovation in areas such as data curation, post-training, or model architectures beyond the transformer, which are all areas I’m excited about and keeping a close eye on. This is aligned with the comments from Aravind Srinivas of Perplexity when asked to opine on this topic.
To wrap this post, I’ll end on another cautionary note by saying that through this fast-moving tide of innovation within AI infrastructure, it is important to stay extra vigilant around claims that are not based on published research, and even those based on peer reviewed materials should not be taken at face value.
Thank you for sharing Janelle! I think the point around bundling, unbundling, etc is particularly interesting due to the importance of data and specific workflows to LLM training and usage. Can imagine the big data players with “data gravity” (hyperscalers, Databricks, Snowflake) ultimately bundling a lot of infra capabilities bc the data you need is there. Can also see the big vertical SaaS players bundling vertical specific AI apps into their products as they have both the workflows and the data. Already seeing some of this with the acqui-hired of Inflection and Adept by the hyperscalers….